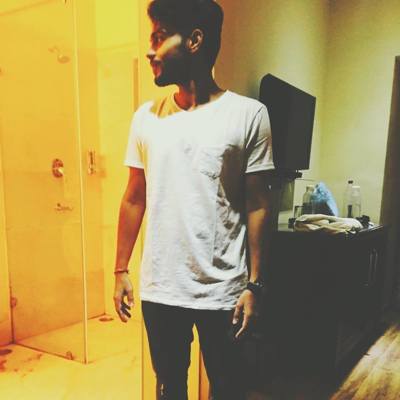
Akshay Bahadur
IC3 Engineer, Yahoo
San Jose, California, United States
Actions
My interest towards computer science sparked when I was working on a women's safety application aimed towards the women welfare in India and since then I have been incessantly working on improving my skills. I stumbled upon machine learning then. I have made several open-source contributions in the field of ML and would continue to do so. I have made successful prototypes like autonomous car, alphabet recognition, cancer classification, gesture recognition using learning models. These prototypes showcase the power of deep learning and how I can help your organization implement learning models to solve business cases. My ambition is to make valuable contribution towards ML community and leave a message of perseverance and tenacity. Currently, I am working as a software engineer at Symantec, India. I am also deeply influenced by literature especially Shakespeare's work.
Minimizing CPU utilization for deep networks
The advent of machine learning along with its integration with computer vision has enabled users to efficiently to develop image-based solutions for innumerable use cases. A machine learning model consists of an algorithm which draws some meaningful correlation between the data without being tightly coupled to a specific set of rules. It's crucial to explain the subtle nuances of the network along with the use-case we are trying to solve. With the advent of technology, the quality of the images has increased which in turn has increased the need for resources to process the images for building a model. The main question, however, is to discuss the need to develop lightweight models keeping the performance of the system intact.
To connect the dots, we will talk about the development of these applications specifically aimed to provide equally accurate results without using much of the resources. This is achieved by using image processing techniques along with optimizing the network architecture.
These applications will range from recognizing digits, alphabets which the user can 'draw' at runtime; developing state of the art facial recognition system; predicting hand emojis, developing a self-driving system, detecting Malaria and brain tumor, along with Google's project of 'Quick, Draw' of hand doodles.
In this presentation, we will discuss the development of such applications with minimization of CPU usage.
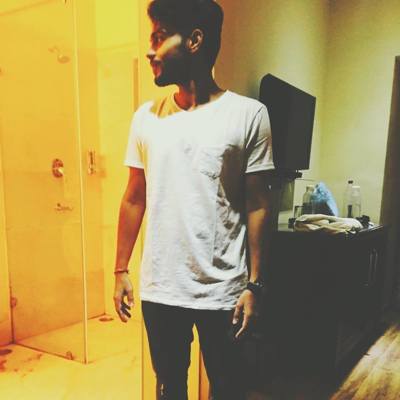
Akshay Bahadur
IC3 Engineer, Yahoo
San Jose, California, United States
Actions
Please note that Sessionize is not responsible for the accuracy or validity of the data provided by speakers. If you suspect this profile to be fake or spam, please let us know.
Jump to top