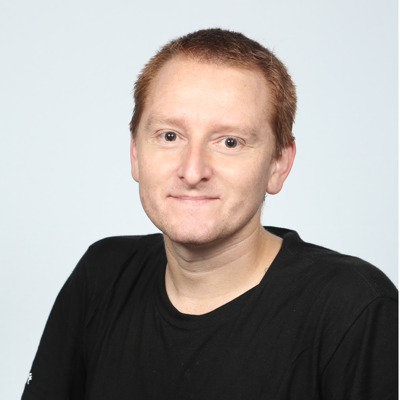
Patricio Cofre
EY Partner and Microsoft MVP/RD
Socio de EY y Microsoft MVP/RD
Santiago, Chile
Actions
Master of Engineering Management, Northwestern University and Master of Engineering Sciences, Catholic University of Chile. Patricio has developed and led projects in analytics for various companies in Latin America. He has specialized in Data Lakes and Artificial Intelligence. Patricio is co-founder of Metric Arts, a Big Data and Analytics specialized firm, recently acquired by EY (Ernst and Young). He is also lecturer at the Catholic University of Chile and Florida University in USA. In 2016 Patricio was recognized as Microsoft Artificial Intelligence MVP and Regional Director.
Master en Ingeniería, Northwestern University (Chicago, USA). Ha liderado proyectos de Analytics para diversas empresas de Latinoamérica. Es co-fundador de Metric Arts (recientemente adquirida por EY). Además, se desempeña como profesor en la Universidad Católica de Chile
Area of Expertise
Topics
Copilot for Power BI en
Power BI has introduced generative AI features that allows you to create reports, create narratives for reports and create enhanced Q&A experiences. This session provides an overview and demos of the capabilities that were introduced for Power BI.
Machine Teaching for Industrial Autonomous Systems en
Machine teaching is a new, complementary approach to machine learning that can be used by those without AI expertise. With machine teaching, a complex problem is broken into individual skills and give the AI important clues about how to learn faster, by focusing on extracting knowledge from experts, rather than only from data.
While traditional reinforcement learning is a time-consuming approach with lots of trial and error, machine teaching accelerates and improves the training process and even allows engineers to reuse the individual steps.
Because companies can’t afford to take critical equipment offline or risk damaging a system while the AI learns, the Reinforcement Learning process takes place in safe and cost-effective simulated environments, that can replicate millions of different real-world scenarios, including edge situations like a sensor failure, so the AI can learn how to adapt.
Machine teaching also makes it easier to understand and audit the autonomous control system’s behavior once it’s been deployed, which is crucial for safety-critical applications.
Machine Teaching para Sistemas Autonomos Industriales en
Machine Teaching es un nuevo enfoque complementario del Machine Learning que puede ser utilizado por quienes no tienen experiencia en IA. Con Machine Teaching, un problema complejo se divide en habilidades individuales y da a la IA pistas importantes sobre cómo aprender más rápido, centrándose en la extracción de conocimientos de los expertos, en lugar de sólo de los datos.
Mientras que el Reinforcement Learning tradicional es un enfoque que requiere mucho tiempo y mucho ensayo y error, Machine Teaching acelera y mejora el proceso de aprendizaje e incluso permite a los ingenieros reutilizar los pasos individuales.
Dado que las empresas no pueden permitirse desconectar equipos críticos o arriesgarse a dañar un sistema mientras la IA aprende, el proceso de Reinforcement Learning se lleva a cabo en entornos simulados seguros y rentables, que pueden reproducir millones de escenarios diferentes del mundo real, incluidas situaciones límite como el fallo de un sensor, para que la IA pueda aprender a adaptarse.
El Machine Teaching también facilita la comprensión y la auditoría del comportamiento del sistema de control autónomo una vez desplegado, lo que es crucial para las aplicaciones de seguridad crítica.
I don't want to talk to you. Can I talk to the chatbot? en
People no longer want to pick up the phone, and talk to a person at your company. They want to communicate with you the same way they communicate with their friends, through text, social media, or a voice assistant.
Reinforcement Learning using Azure Cognitive Services en
Reinforcement learning (RL) is an area of machine learning (ML) concerned with how software agents ought to take actions in an environment in order to maximize the notion of cumulative reward.
Reinforcement learning differs from supervised learning in not needing clean labelled input/output pairs be presented, and in not needing sub-optimal actions to be explicitly corrected. Instead the focus is on finding a balance between exploration (of uncharted territory) and exploitation (of current knowledge).
Azure now offers Personalizer, a new cloud based cognitive service that allows your application to choose the best experience to show to your users, learning from their collective real-time behavior.
Getting started with prompt flow en
Prompt flow is a powerful feature that simplifies and streamlines the Prompt Engineering process for LLM-infused applications. It enables users to create, evaluate, and deploy high-quality flows with ease and efficiency. In this session you'll learn how to enable, create and develop your first prompt flow, test and evaluate it, then deploy it to production.
Assessing and mitigating fairness issues in AI en es
Fairness of AI systems is one of the key aspects in building trust in the use of AI, and thus unlocking its great potential. Societal and technical aspects shape who might be harmed by AI systems and how. There are many complex sources of unfairness and a variety of societal and technical processes for mitigation.
In this session, a demo for credit-card default models will be shown. Fairness-related harms may arise when the model makes more mistakes for some groups of applicants compared to others. These predictions are sometimes used to automatically reject or accept an application, directly impacting both the applicant and the organization.
The use metrics and algorithms that considers the AI system's broader societal context will be explained, so data scientists can use them to improve fairness of AI systems.
Evaluando y mitigando problemas de equidad en la IA en es
La equidad de los sistemas de IA es uno de los aspectos clave para generar confianza en el uso de la IA y así poder liberar su gran potencial. Aspectos sociales y técnicos determinan quién y cómo puede resultar perjudicado por los sistemas de IA. Hay muchas fuentes de inequidad y una variedad de procesos sociales y técnicos para su mitigación.
En esta sesión se presenta una demo de modelos de no-pago de tarjetas de crédito. Los perjuicios relacionados con la equidad pueden surgir cuando el modelo comete más errores para algunos grupos de solicitantes en comparación con otros. Estas predicciones se utilizan muchas veces para rechazar o aceptar automáticamente una solicitud, lo que repercute directamente tanto en el solicitante como en la organización.
El uso de métricas y algoritmos que toman en cuenta el contexto social amplio del sistema de IA será explicado, de modo que los científicos de datos puedan utilizarlos para mejorar la equidad de los sistemas de IA.
Multivariate Anomaly Detection en es
The new multivariate anomaly detection APIs in Anomaly Detector further enable developers to easily integrate advanced AI of detecting anomalies from groups of metrics into their applications without the need for machine learning knowledge or labeled data. Dependencies and inter-correlations between different signals are now counted as key factors. The new feature protects your mission-critical systems and physical assets, such as software applications, servers, factory machines, spacecraft, or even your business, from failures with a holistic view.
Detección de Anomalías Multivariadas en es
Las nuevas APIs de detección de anomalías multivariadas permiten a los desarrolladores integrar fácilmente la IA avanzada de detección de anomalías a partir de grupos de métricas en aplicaciones sin necesidad de conocimientos de machine learning o datos etiquetados. Las dependencias y correlaciones entre diferentes señales se cuentan ahora como factores clave. Esta nueva función puede proteger sus sistemas y activos físicos de misión crítica, como aplicaciones de software, servidores, máquinas de fábrica, naves espaciales o incluso su negocio, de los fallos con una visión holística.
Global AI Night Latinoamérica 2021 Sessionize Event
Dev Around The Sun Sessionize Event
Global Azure Virtual Sessionize Event
Global AI Community - On virtual tour Sessionize Event
Please note that Sessionize is not responsible for the accuracy or validity of the data provided by speakers. If you suspect this profile to be fake or spam, please let us know.
Jump to top