Session
Exploring and Understanding Databricks Lakehouse Monitoring
Data lakes have become a core part of our data solutions. We push immense amounts of data, from various sources into our lakes on a daily, hourly, and even minute by minute, second by second basis. This data is constantly evolving, and must be nurtured and explored for us to understand it. Our applications consuming this data must be able to adapt as the data grows and evolves, in particular when we are working with Machine Learning Models.
To achieve this we must understand our data integrity, be able to visualise and alert on how the data changes over time, but what tooling can we use to achieve this? Databricks have recently announced a new feature that solves this problem: Databricks Lakehouse Monitoring. Databricks Lakehouse Monitoring lets you create time granular observations and set up custom metrics on your Data Lake. In particular, Databricks Lakehouse Monitoring allows you to analyse the statistical distribution of the data, and look for drift between the current data and a known baseline. It allows you to see when ML model inputs and predictions are shifting over time, and identify model performance trends.
This all sounds great, and a solution to our problem, but how good is Databricks Lakehouse Monitoring? What does and doesn’t it give us? How much will it cost? In this session we will explore Databricks Lakehouse Monitoring, digging into its key features, analysing how they can work for us, so we can better understand our data integrity.
By the end of this session you will have a better understanding of Databricks Lakehouse Monitoring, how to implement it, and if it is a good fit for you.
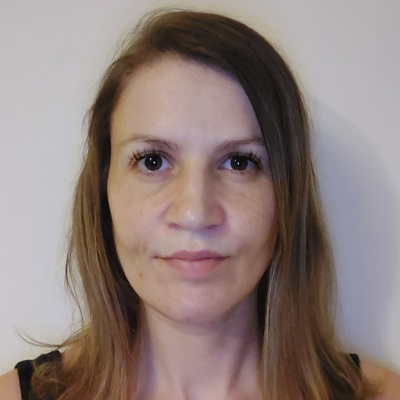
Anna-Maria Wykes
Microsoft Data Platform & AI MVP | Data & AI RSA (Resident Solution Architect) and Consultant
Bristol, United Kingdom
Links
Please note that Sessionize is not responsible for the accuracy or validity of the data provided by speakers. If you suspect this profile to be fake or spam, please let us know.
Jump to top